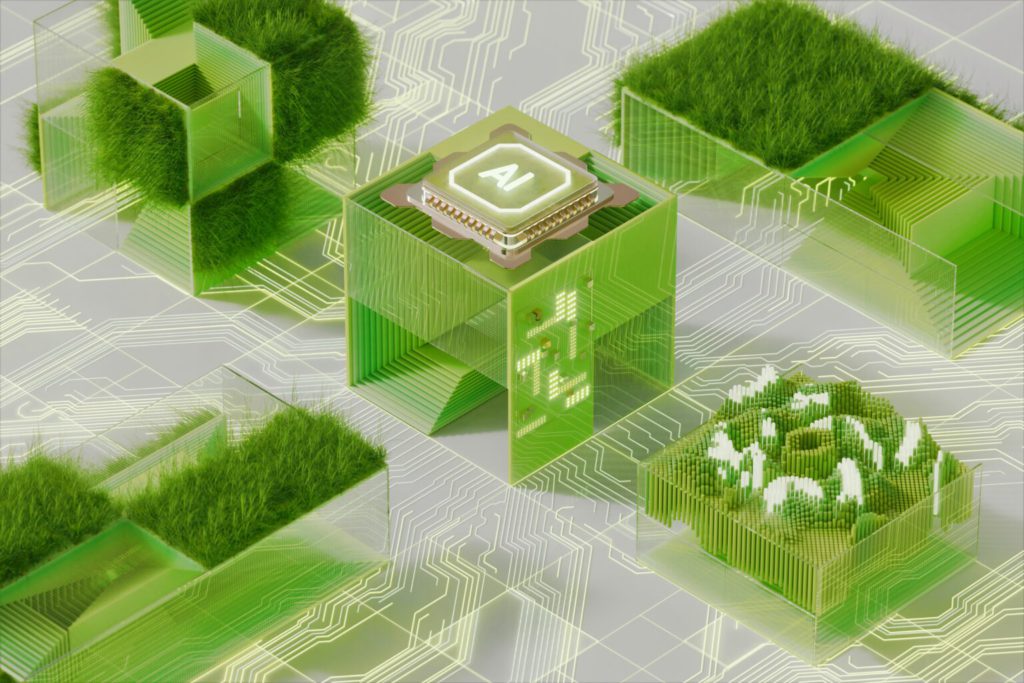
Following the leaps in Generative AI (GenAI), you may think it’s a prevalent technology. Media and analysts are reporting widely on its many valuable business applications. But the total transformation we were promised is happening only gradually. Why? Because implementing AI responsibly means mitigating risks around ethics, sustainability, and cybersecurity in the first place. Also, because generative AI opens new possibilities but does not cover for all other existing AI technologies.
Companies need to make strategic decisions or organizational changes before using GenAI at scale. In our case, we launched the AI Hub at Schneider Electric even before GenAI was brought to market. But we still decided to instate a review process with internal and external stakeholders to detect early application opportunities for this technology. Looking across all functions, we selected more than 200 potential use cases. Not all of them passed the responsible AI framework evaluation, and we abandoned those with only medium or uncertain value for customers. By now, the first applications we built have started to increase internal productivity and enhance our offers. Most of them support the core mission of our company – making a positive impact on the planet by equipping our customers and partners for their sustainability journey.
How can GenAI support sustainability?
In the recently launched Resource Advisor Copilot, we employ GenAI-based Natural Language Processing (NLP) technology to offer a convenient digital companion. Through a chat interface, you can ask Copilot to retrieve real-time data, perform enhanced analytics and visualization, or tap into our industry knowledge and system information to provide decision support and optimization hints. With it, we wanted to help customers move toward carbon neutrality by providing answers to their questions like:
- What are the total scope 1 emissions for our US site over the past six months?
- What does scope 3 emissions mean?
- How much did we spend on electric power in 2022?
- Can you detail a decarbonization plan to achieve my emissions goals in the next five years?
But GenAI isn’t the only AI technology that can support companies with their ESG commitments. Let’s take a look at some others.
Machine learning
Machine learning (ML) allows us to analyze large datasets, identify patterns, and make predictions. It can also automate predictive analysis and make data-based decisions quicker. ML directly supports the transition to renewable energy — one of the most effective decarbonization strategies. It does this notably by working on the demand side of the demand/supply equation. It enables to optimize the usage of renewable energy by analyzing multiple data sources and detecting inefficiencies to optimize energy usage.
For example, Ecostruxure Microgrid Advisor software connects to your distributed energy resources. It automatically forecasts and optimizes how and when to consume, produce, and store energy. With this solution, our customers like Lidl Finland and Citycon shopping center in Lippulaiva got closer to net zero. Here we used a ML algorithm that constantly analyzes data from energy generators, EV charging stations, batteries, back-up generators, HVAC systems, lighting systems, UPS, combined heat and power (CHP), and utility metering.
Deep Learning
Deep Learning (DL) is a subset of ML. It uses neural networks with multiple layers to extract complex features from data. DL works in various domains, like demand response optimization, renewable energy forecasting, and energy grid management. These areas are seeing the biggest progress in AI industrialization.
DL can help optimize energy use in a new or a newly instrumented building with limited or low-quality data available at the start. In a recent paper titled “Cold Start Methods for Building’s Energy Consumption Forecasting”, our data scientists worked with IMT Atlantique to apply DL in Smart Energy Management Systems (SEMS) — reducing energy waste in the early building deployment phase. SEMS are crucial for minimizing emissions, optimizing energy consumption in buildings, and creating management strategies. The key to unlocking their value is accurate forecasting.
How can you train forecasting models for real estate development with little data? A Cold Start approach uses historical data from buildings with similar characteristics — before applying this knowledge to the new task. The result is reduced energy waste from the get-go. This is good for the environment, saves money, and makes buildings more valuable.
This article appeared in Schneider Electric and has been published here with permission.
Creating responsible AI solutions
Traditional ML and DL require less computation than generative AI and save a lot of energy. The final verdict whether AI is good for the planet depends on every single use case that businesses and organizations decide to implement. Our approach is that the energy saved must outweigh the energy needed to power an AI model. With a dedicated decision framework to make these kinds of choices, we ensure this technology is responsible, ethical, and serves a meaningful purpose. If you want to learn more about sustainability solutions for your building, contact us today!